Can artificial agents do research on the effects of humans’ interactions with artificial agents?
The emergence of AI-powered artificial agents, such as service robots and chatbots, is expected to transform many sectors, including retail, hospitality, and healthcare. And as these agents become more prevalent, understanding their impact on us humans becomes important. Indeed, this is a main rationale behind the project Interactions with Artificial Humans in which I am involved and in which I have had the chance to conduct several studies. But the time has come to ask myself this: why am I doing all the things I am doing in this project? If artificial agents are becoming more humanlike, more intelligent, and more autonomous, why can’t they do the research themselves? Why do people like myself need to be involved? Can these artificial agents serve us also when it comes to studying the effects of artificial agents? Can they conduct the very research that examines their influence on us? Here, I want to offer some reflections on this, particularly when it comes to what is needed in academic research and when using experimental methods.
There must be questions to examine
As a point of departure, research needs questions to be answered. Can an artificial agent come up with relevant questions? And can it do this in such way that a study answering the questions can be published as an article in an academic journal? Nowadays, human researchers need to think carefully about such questions if they want to publish a study. The question should not only be relevant in various ways; it should also be more or less unanswered by other researchers. And the variables involved in the question should fit into theoretical frameworks stemming from others’ collective efforts in previous research. To develop such questions can indeed be difficult even for a seasoned human researcher. Yet, an artificial agent can do this if it has access to a massive volume of existing research, because many studies comprise the researchers’ explicit thoughts about what remains to be done. Typically, this is delivered in the end of a paper under headings such as “Limitations and suggestions for further research” or “Future research directions”. So, an artificial agent with a large language model has plenty of material to use to formulate research questions. ChatGPT,
for example, is doing a relatively decent job when I ask it about the most important questions that research should address in the area of the effects of artificial agents on humans.
There must be testable hypotheses linked to theory
Next, questions need to be converted into hypotheses. Typically, a hypothesis is an empirically testable proposition about some behavior, mind state or relationship, usually based on theory, and it is stating an expected outcome. Also this can be challenging for a human researcher. What is particularly challenging is to present a hypothesis so that conceptual arguments make it clear why whatever is proposed in the hypothesis is likely to happen. Consequently, many published studies (and many studies that are rejected in a review process) are not optimal in this respect – the “why” aspect is missing [1]. It is common in an academic journal article, for example, that the author builds the basis for a hypothesis by citing researchers who have empirical evidence for what a hypothesis states. That is to say, the author writes that researcher so and so found in a previous study that X impacts Y, and therefore the hypothesis in the current study is that X influences Y (i.e., H1: X has a positive influence on Y). An artificial agent that has been exposed to many previous studies, however, may be able to do this better, namely to explicitly articulate in conceptual terms why X is likely to have an impact on Y. Hopefully, an artificial agent may also be better at avoiding formulating hypotheses that are well-nigh tautologies (e.g., the more useful a service robot is perceived to be, the more likely it is that it will be used).
More complexity is added given that a hypothesis is supposed to be testable. This requires two types of knowledge (already when a hypothesis is formulated). First, there is a need to know how to translate what the hypothesis states into a setting in which it can be tested with empirical data. How this should be done is seldom stated in the method literature, and it typically requires creative skills from the researcher. Example: if you want to test, in an experiment, that a cognitively challenging task impedes people’s attention to what they can see in front of themselves, it is not obvious that participants should be asked to count the number of passes in a basketball game while you, the experimenter, designs the test situation so that a gorilla is appearing in the midst of the game. Yes, this experiment has been made. The history of experiments is indeed full of such creative translations from what is conceptual to what is a setting in which hypotheses can be tested [2]. Can an artificial agent really do this? Maybe it can, if it has access to a rich body of text about what has been done in previous experiments.
Second, there is a need to know what specific statistical test that should be used later on, which typically means to make selections from a vast pool of available tests. For an experiment, however, in which the core analysis is to compare if groups of participants who receive different treatments react with different response levels, a limited number of tests are used frequently by human researchers (such as t-tests and ANOVAs). Thus, thousands of experiments exist with these tests. To apply them in a new experiment, then, may not be so tricky for an artificial agent with access to existing research.
There must be data collection from participants
To make an experiment happen requires additional skills, particularly with respect to recruiting participants who can be exposed to what is manipulated in the experiment. If, for example, a service robot in a field experiment should interact in a store with customers (who do not know that they take part in an experiment), the researcher needs to have access to a store in which the robot can be placed while the experiment is conducted. Someone needs to talk to the retailer about this. And not every retailer is likely to say yes to have its customers exposed to various robot treatments. Once the robot is in the store, however, it can be programmed to execute experimental protocols. It can randomly assign participants to different conditions, ensure consistent delivery of experimental treatments, and systematically record responses. Indeed, it can collect data through its sensors, including visual, auditory, and behavioral responses from customers. For instance, a service robot in a retail setting can expose customers to various versions of a treatment, such as different types of greetings (e.g., “Hi human!” vs. “Hi!”) and it can record facial expressions and what the customer says – spontaneously or as a responses to standardized questions. Maybe this type of data collection can be more consistent and comprehensive than human researchers’ efforts, which would reduce biases and errors.
In other cases, participants will indeed know that they are participating in a study. But before the study can be conducted, they must be informed about the study and persuaded into taking part. It is not obvious how an artificial agent could accomplish this in a setting in which humans are the unit of analysis. If other entities than humans are to be examined, however, it may be easier. For example, a group of researchers have created a Robot Scientist that can carry out microbal growth experiments in a laboratory [3]. What is becoming increasingly common in experiments with human participants is that researchers collect data online from participants who are part of a panel (e.g., Amazon’s MTurk) and who are paid for
participating in studies. In such cases, the researcher orders a specific number of participants (and pays them) online. The researcher never meets them face-to-face; they could be anywhere while they participate in a study. Typically, they are given a link to click on – a link leading to a webpage where stimuli are shown, which is followed by questionnaire items to capture their reactions. Who knows, maybe clever artificial agents can be taught how to set up studies like this. In any event, what they can do already now is this: they can generate questionnaire items so that the measure of a specific variable conforms to the measurement standards in a research field.
There must be an analysis of the data
A typical experiment results in comparing the levels of responses for participants who received different treatments. For example, if there are two versions of the treatment (e.g., a retail service robot’s greeting is “Hi Human!” vs. “Hi!”), and if the response is customer satisfaction (assessed with a scale ranging from 1 to 10), the experimenter computes the mean response level for each participants and, in the next step, the levels are compared between the treatments. Then, in the final step, the experimenter tests if the difference in levels is statistically significant. Once the data have been collected, this analysis part is relatively mechanical – it is the same in basically every experiment that has ever been published. So
there is a lot of material available for teaching artificial agents how to do this.
There must be a text about the study
Today, few human researchers would stop when they see the results of an experiment. The final step is perhaps the most labor intense: the study needs to be described in a coherent text – and it is this text that eventually can become a published journal article. A less exciting aspect of such texts, if they are about a typical experiment to assess the impact of artificial agents on human users or customers, is that they invariably follow the same basic structure (introduction, theory, method, analysis and results, discussion). The language is typically similar, too: it is impersonal, often in the passive voice, and definitely not in a literary fiction style. During recent years, Natural Language Generation (NLG) technologies have made
significant strides, enabling AI-based tools to draft coherent texts consistent with academic standards. Advanced models can most likely also mimic the format required by a specific academic journal. Already today, and with the right prompts, tools such as ChatGPT can produce very convincing journal article texts.
So, should we retire the human researchers?
Given everything that the artificial researchers can do already, can we let them loose now? Can we ask them to do research on how we humans react to artificial agents? Well, as indicated, there are some activities that would be difficult for them, particularly the practicalities of recruiting human participants to a study. Reacting to reviewers’ comments in a peer review process may be tricky, too. And if artificial agents become self-aware in the same way as humans are, and if they develop ideas that they are unique, special and superior (as we humans tend to do), one may foresee that there can be non-flattering results in studies of the effects of artificial agents that artificial researchers would prefer not to publish.
But let’s say that many such issues can be solved. And for those issues that cannot be solved, maybe we humans can serve as technicians who serve the artificial researcher. If this is possible, would it result in better research? Some human researchers seem to think so. If all aspects of the scientific process can be automated, they say, it would improve the accuracy of experiments, increase the pace of discovery, and remove the boring, repetitive tasks from the human researcher [4].
Well, there is one more issue that needs attention when artificial agents become researchers:
the authorship issue. No research is published in serious journals without an author. And we humans have built an increasingly complex system in which journal article authorship is determining if an individual will be allowed to defend a thesis, get tenure, obtain a grant, and become a full professor. To date, these activities have been reserved for humans. So if the author is non-human, it calls for some fundamental re-thinking of the social system we have created for scientific endeavors.
References
[1] Sutton, R. I., & Staw, B. M. (1995). What theory is not. Administrative Science Quarterly, 40 (3), 371-384.
[2] Söderlund. M. (2018). Experiments in Marketing. Lund: Studentlitteratur.
[3] Soldatova, L. N., Clare, A., Sparkes, A., & King, R. D. (2006). An ontology for a Robot Scientist. Bioinformatics, 22 (14), e464-e471.
[4] Sparkes, A., Aubrey, W., Byrne, E., Clare, A., Khan, M. N., Liakata, M., & King, R. D. (2010). Towards robot scientists for autonomous scientific discovery. Automated Experimentation, 2, 1-11.
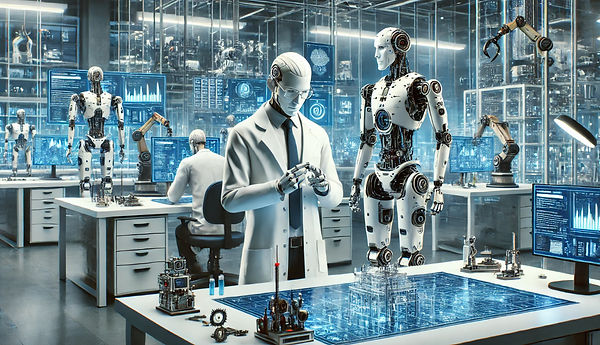
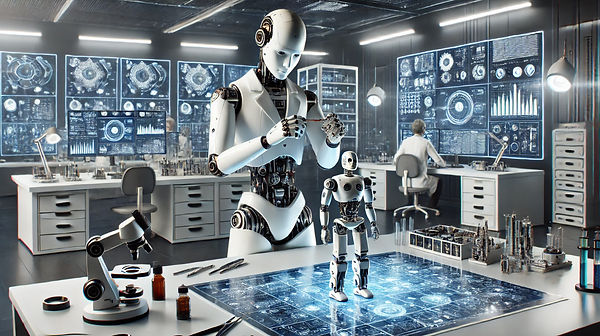